A Pioneer in Intelligent Tutoring Systems (1997-2020+)
Core Concept and Innovation
AutoTutor was an intelligent tutoring system developed at the University of Memphis that engaged students in natural language conversations to facilitate learning.
Its key innovation was the ability to hold meaningful educational dialogues while adapting to students’ cognitive and affective states.
The system used animated agents that communicated through synthesized speech, facial expressions, and gestures.
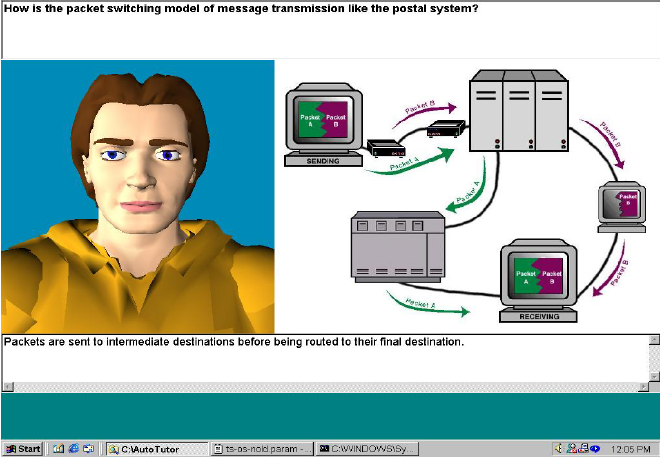
Technical Capabilities
- Natural language processing for student dialogue
- Emotion recognition through facial expressions, speech, and body posture
- Conversational strategies modeled after human tutors
- LSA (Latent Semantic Analysis) for understanding student responses
- Curriculum scripting for different subject domains
- Adaptive dialogue management through the Dialog Advancer Network (DAN)
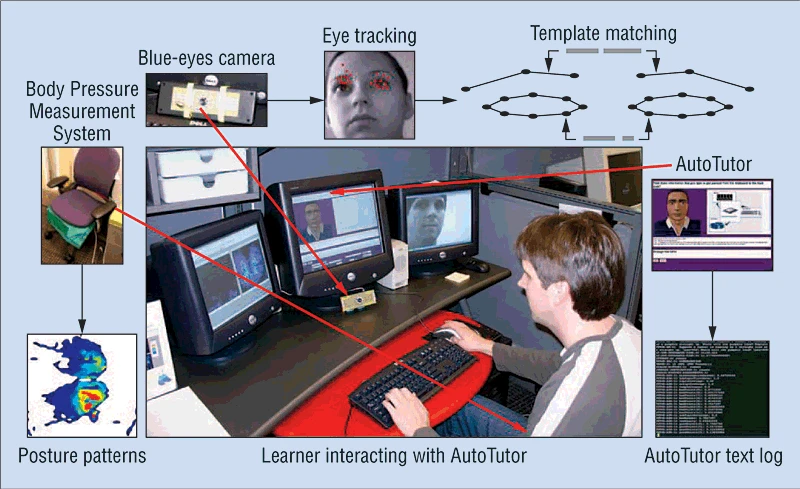
Evolution and Variants
The original AutoTutor spawned numerous specialized versions:
- AutoTutor-Lite: Simplified version with broader subject matter compatibility
- AutoTutor-3D: Enhanced visual capabilities
- AutoTutor-Affect-Sensitive: Emotion-aware version
- DeepTutor: Advanced physics tutoring
- GuruTutor: Specialized for biology
- Operation ARIES: Focus on scientific reasoning
- MetaTutor: Self-regulated learning
- CSAL: Adult literacy tutoring
Key Contributors and Leadership
The project was primarily led by:
- Dr. Arthur C. Graesser (Principal Investigator)
- Dr. Xiangen Hu
- Dr. Stan Franklin
- Dr. Rosalind Picard (MIT collaboration)
- Zhiqiang Cai (Chief Programmer)
Impact and Effectiveness
AutoTutor demonstrated significant educational benefits:
- Learning gains of approximately 0.8 standard deviations above control groups
- Performance comparable to expert human tutors
- Successfully implemented across multiple domains (computer literacy, physics, critical thinking)
- Passed “bystander Turing tests” for natural conversation quality
- Led to dozens of research publications and multiple funded projects
Historical Significance and Legacy for Modern AI
AutoTutor was groundbreaking in several ways that influenced current AI systems:
- Natural Language Interaction: AutoTutor was one of the first systems to demonstrate successful natural language tutoring at scale, paving the way for modern conversational AI in education. Its work on dialogue management and natural language understanding helped establish foundations that current LLMs build upon.
- Multimodal Interaction: The system’s integration of facial expressions, speech, and gesture recognition was ahead of its time and mirrors current efforts in multimodal AI systems.
- Adaptive Learning: AutoTutor’s ability to adjust to student responses and emotional states presaged modern adaptive learning systems and emotional AI.
Evolution into Current AI Systems
AutoTutor’s influence can be seen in modern AI tutoring applications:
- The emphasis on natural dialogue is reflected in current LLM-based tutoring systems
- Its work on emotional recognition and response has influenced modern affective computing
- The focus on pedagogical agents has evolved into current AI teaching assistants
- The system’s adaptive response mechanisms are similar to those used in modern personalized learning platforms
Today’s AI tutoring systems, while more advanced in their language processing capabilities thanks to large language models, still grapple with many of the same challenges AutoTutor addressed: maintaining coherent educational dialogues, adapting to student needs, and providing effective feedback.
AutoTutor’s research into these areas laid important groundwork for current developments in AI-powered education.
The project stands as a significant milestone in the development of intelligent tutoring systems and demonstrates how early work in specialized AI systems contributed to the broader evolution of artificial intelligence in education.